Linghan ZHANG
张凌寒
About the author Zhang Linghan, Ph.D., Professor at the Institute of Data Law and doctoral supervisor, China University of Political Science and Law, visiting scholar at Cornell University.
She has published extensively on cyberspace law and algorithmic governance. She is the author of “Research on Tort Liability of Online Virtual Property”and “Governing Power : Regulation of Algorithms in the Age of Artificial Intelligence.” She is a member of the UN High-Level Advisory Body on AI, an expert member of the Information and Communication Science and Technology Committee of the Ministry of Industry and Information Technology, and expert member of the Cybersecurity Legal Advisory Committee of the Ministry of Public Security. She has participated in the legislative advisory work on relevant laws related to algorithms regulation, platform governance, data security and artificial intelligence governance in China for years.
关于作者张凌寒,法学博士,中国政法大学数据法治研究院教授,博士生导师,曾为康奈尔大学访问学者。研究方向为民商法、数据法,近年来专注人工智能(算法)、数据和平台治理等法律问题的研究。
她已出版专著《网络虚拟财产侵权责任研究》,《权力之治:人工智能时代的算法规制》。担任联合国高级别人工智能咨询机构专家,工信部信息通信科学技术委员会专家委员、中国信息安全法律委员会专家委员,曾参与我国多项人工智能和算法、数据和平台治理相关法律法规的立法咨询工作。
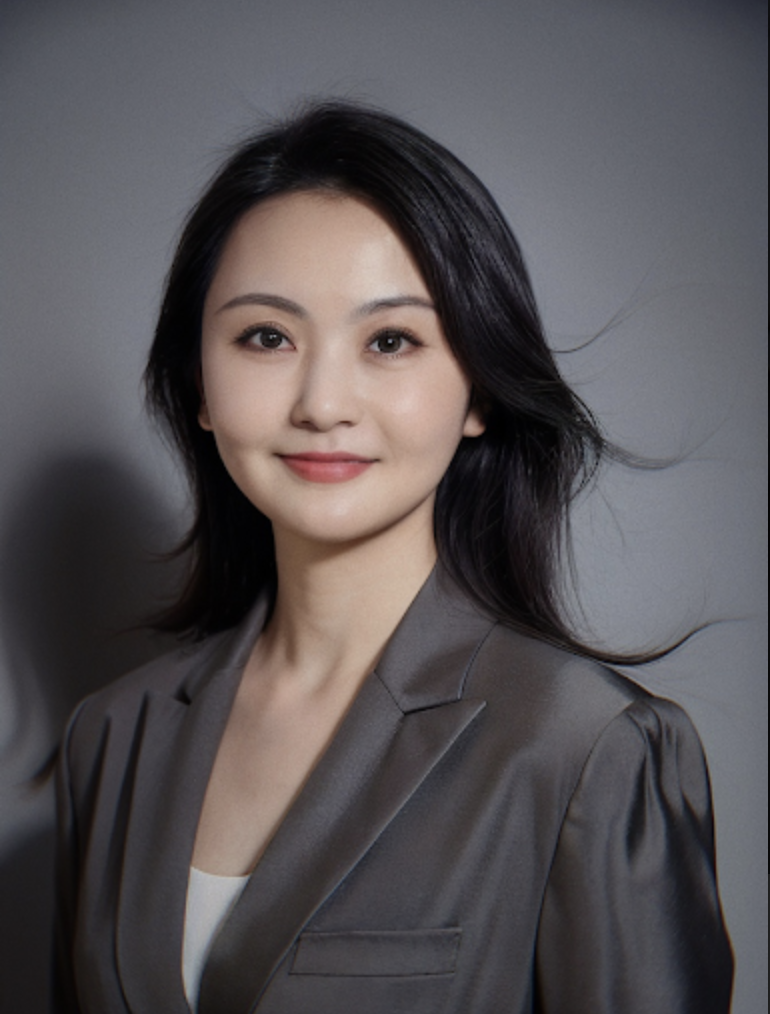
The following excerpt is a translation of the paper titled “From Traditional Governance to Agile Governance: Paradigm Innovation in the Governance of Generative Artificial Intelligence” (张凌寒,于琳丨从传统治理到敏捷治理:生成式人工智能的治理范式革新), co-authored by Linghan Zhang and Lin Yu.
▶ Cite Our Translation
Concordia AI. “Linghan Zhang — Chinese Perspectives on AI Safety.” Chineseperspectives.ai, 29 Mar. 2024, chineseperspectives.ai/Linghan-Zhang.
▶ Cite This Work
张凌寒 & 于琳(2023). 从传统治理到敏捷治理:生成式人工智能的治理范式革新. 电子政务(09),2-13.
Translation
(II) Evolution of Risks: Uncertainty of Risks Extends from External to Internal
With the advent of generative AI (artificial intelligence), represented by ChatGPT, the uncertainty of risks has extended from external to internal. Based on whether the risks are endogenous, AI risks can be divided into external risks and internal risks. Generally, the potential societal risks of emerging technologies, i.e., external risks, are highly uncertain. This uncertainty often relates to the development pattern of the technology, which always undergoes a process from inception to improvement and maturity, and external risks only gradually manifest during this process. This uncertainty is not only related to the development patterns of emerging technologies but also relates to AI’s strong human-machine interaction and unexplainable reasoning capabilities. The strong human-machine interaction of generative AI makes external risks highly uncertain due to their unpredictability, while its reasoning capabilities further extend this uncertainty from external to internal.
(II) Evolution of Risks: Uncertainty of Risks Extends from External to Internal
With the advent of generative AI (artificial intelligence), represented by ChatGPT, the uncertainty of risks has extended from external to internal. Based on whether the risks are endogenous, AI risks can be divided into external risks and internal risks. Generally, the potential societal risks of emerging technologies, i.e., external risks, are highly uncertain. This uncertainty often relates to the development pattern of the technology, which always undergoes a process from inception to improvement and maturity, and external risks only gradually manifest during this process. This uncertainty is not only related to the development patterns of emerging technologies but also relates to AI’s strong human-machine interaction and unexplainable reasoning capabilities. The strong human-machine interaction of generative AI makes external risks highly uncertain due to their unpredictability, while its reasoning capabilities further extend this uncertainty from external to internal.
原文
(二)风险演变:风险不确定性由外部延伸至内部
以ChatGPT为代表的生成式人工智能出现后,风险的不确定性由外部向内部延伸。依据风险是否具有内生性,人工智能风险分为外部风险和内部风险。一般而言,新兴技术的潜在社会风险也即外部风险普遍具有高度不确定性,这种不确定性往往与技术发展规律有关,技术的发展总是需要经历一个从产生到完善再到成熟的过程,外部风险只能在这一过程中逐渐显露。生成式人工智能的风险不确定性不仅与新兴技术发展规律有关,还与自身的强人机互动性和无法解释的推理能力有关。生成式人工智能的强人机互动性使外部风险由于难以预知而具有高度不确定性,而其自身的推理能力更是将这种不确定性由外部延伸至内部。
(二)风险演变:风险不确定性由外部延伸至内部
以ChatGPT为代表的生成式人工智能出现后,风险的不确定性由外部向内部延伸。依据风险是否具有内生性,人工智能风险分为外部风险和内部风险。一般而言,新兴技术的潜在社会风险也即外部风险普遍具有高度不确定性,这种不确定性往往与技术发展规律有关,技术的发展总是需要经历一个从产生到完善再到成熟的过程,外部风险只能在这一过程中逐渐显露。生成式人工智能的风险不确定性不仅与新兴技术发展规律有关,还与自身的强人机互动性和无法解释的推理能力有关。生成式人工智能的强人机互动性使外部风险由于难以预知而具有高度不确定性,而其自身的推理能力更是将这种不确定性由外部延伸至内部。
Due to the strong human-machine interaction of generative AI, its external risks are difficult to predict. Previously, whether in the era of professionally-produced content or user-generated content, platforms had strong control over the creation, publication, and dissemination of information content, with the main responsibility for information content security borne by platforms. Article 47 of the Cybersecurity Law was the first law or regulation to clarify the legal responsibility of network operators for managing user information publication, and subsequent regulations including the Provisions on Ecological Governance of Network Information Content and Opinions on Further Consolidating the Responsibility of Website Platforms as the Information Content Management Entity further detailed the responsibility for platforms as the main entity for information content security.
基于生成式人工智能的强人机交互性,生成式人工智能的外部风险难以预知。此前,无论是在专业生产内容时代还是用户生产内容时代,平台对信息内容的制作、发布、传播都具有较强的控制力,信息内容安全的主体责任主要由平台承担。《网络安全法》第四十七条首先在法律层面明确了网络运营者对用户信息发布的管理责任,《网络信息内容生态治理规定》《关于进一步压实网站平台信息内容管理主体责任的意见》又进一步细化了平台的信息内容安全主体责任。
However, in the era of AI-generated content, generative AI service providers lack the ability to control the input end. Unlike traditional AI products or services that are delivered in one direction, from provider to user, generative AI products or services are provided through interaction with users. Concretely, users input their demands, and the model generates corresponding results based on the user's input. What content is generated depends largely on the specific demands of the user. The "user input + machine output" content generation pattern means that even if service providers fulfill their compliance obligations in research and development at the front end, users can still breach compliance at the input end. Although service providers usually take preventive measures to restrict user input behavior, the actual results are not always satisfactory. For example, although OpenAI explicitly prohibits the generation of malicious software in its usage policy, researchers found that users can deceive ChatGPT into writing code for malicious software through misleading prompts. The platforms’ difficulty in controlling the input end means that the content the users input, the results the model generates, and the impact of the generated results are all unknown.
然而,在人工智能生成内容时代,生成式人工智能服务提供者不具备控制输入端的能力。区别于传统人工智能产品或服务在提供方式上的单向性,生成式人工智能产品或服务的提供是通过与用户交互完成的,具体表现为用户输入需求,模型根据用户输入的内容生成相应结果,生成何种内容在很大程度取决于用户输入的具体需求。“用户输入+机器输出”的内容生成方式意味着,即便服务提供者在前端依法履行了研发合规义务,用户依然能够在输入端打破合规性。虽然服务提供者通常会采取事前预防措施对用户的输入行为作出一定限制,但实际效果不尽如人意。例如,虽然OpenAI在使用政策中明确禁止生成恶意软件,但研究人员发现,用户依然可以通过输入提示欺骗ChatGPT为恶意软件应用程序编写代码。平台难以实现对输入端的控制,意味着用户端输入何种内容,模型生成何种结果,生成结果又将产生何种影响均系未知。
Based on the reasoning capability of generative AI, its internal risks are difficult to predict. Starting from ChatGPT, the GPT series began to possess reasoning capabilities, and even the ChatGPT R&D team cannot explain the reasons behind the emergence of such capabilities. This has, to some extent, changed the nature of the algorithmic "black box" problem. The traditional meaning of the algorithmic "black box" is essentially a technological "black box," characterized by the technical principles being known only to certain people, while remaining unknown to others – namely, regulatory authorities and the public society. However, generative AI, represented by ChatGPT, has to some extent destroyed the traditional societal recognition and understanding of the algorithmic "black box." The essence of the algorithmic "black box" problem has shifted from "information asymmetry between people" to "humanity’s collective ignorance in the face of strong artificial intelligence."
基于生成式人工智能的推理能力,生成式人工智能的内部风险难以预知。从ChatGPT开始,GPT系列开始具备推理能力,即便是ChatGPT的研发团队也无法解读这种能力出现的原因,这在一定程度上改变了算法“黑箱”问题的本质。传统意义上的算法“黑箱”本质上为技术“黑箱”,表现为技术原理仅为部分人所知,而另一部分人不得而知。这里的“另一部[分]人”主要是指监管部门和社会公众。然而,以ChatGPT为代表的生成式人工智能在一定程度上打破了社会对算法“黑箱”的传统认知与理解。算法“黑箱”问题的本质由“人与人之间的信息不对称”转变为“人类在强人工智能面前的共同无知”。
(III) Adaptive Governance Mechanism: Distinguishing Known and Unknown Risks, Emphasizing Prevention and Response of Risks from Generative AI
The unknowns of generative AI risks do not mean that any particular risks it poses are impossible to predict. External risks will gradually become apparent in the process of the technology's popularization and application. At least, in the current stage, people have already gained knowledge of some application risks through the frequent use of generative AI. The DeepMind team has determined that there are 21 existing risks of large models, categorizing them into six risk areas: discrimination, exclusion, and toxicity; information hazards; misinformation harms; malicious uses; human-machine interaction harms; and environmental and socioeconomic harms. However, there are still unknown risks that are difficult to predict due to the unexplainable reasoning capabilities of generative AI and the unpredictability of technological and industrial development trends. Generative AI governance should differentiate known risks from unknown risks according to the predictability of risks, formulate targeted governance plans accordingly, and establish an adaptive governance mechanism for risks.
(三)适应性治理机制:划分已知与未知风险,预防与应对并重生成式人工智能的风险
未知性并不意味着由其引发的任何风险均不可预知,外部风险是会在技术的普及与应用过程中逐渐显露,至少现阶段人们在频繁应用生成式人工智能的过程中,已经获知了一些应用风险。DeepMind团队确定了大模型现存的21个风险,并将这些风险总结为6类风险领域,即歧视、仇恨言论和排斥、信息危害、错误信息危害、恶意使用、人机交互的危害以及环境和社会经济方面的危害。但由于生成式人工智能无法解释的推理能力以及不可预知的技术走向和产业发展趋势,尚存在难以预测的未知风险。生成式人工智能治理应当依照风险的可知性,划分已知风险与未知风险,有针对性地制定治理方案,建立风险适应性治理机制。
For unknown risks, establish a comprehensive response mechanism for during and after incidents. For risks occurring at the foundation model layer and specialized model layer, technical developers should be required to take emergency remedial measures, such as taking the model offline for repairs and suspending the model, to prevent more significant damage. They should also promptly fulfill obligations to notify users (including companies and individuals) and report to regulatory authorities. In addition, since foundation models not only provide model application services to end users but also offer pre-trained large model products to downstream companies in the industry, when major security incidents occur, the foundation model service provider should be required to immediately cease the provision of products to downstream companies. Given the general and empowering nature of foundation models, suspending a foundation model would have a ripple effect on the system. Therefore, the foundation model should be promptly restored to normal operation after repairs.
对于未知风险,建立完备的事中事后应对机制。针对基础模型层和专业模型层发生的风险事件,应当要求技术研发者立即采取离线修复、模型停运等应急补救措施,防止损害进一步扩大,并及时履行对用户(包括企业和个人)的告知义务和对监管部门的报告义务。此外,由于基础模型不仅面向终端用户提供模型应用服务,还面向产业下游企业提供预训练大模型产品。当发生重大安全事件时,还应当要求基础模型服务提供者立即停止对下游企业的产品供应。鉴于基础模型的通用性与赋能性,基础模型停运带来的影响将是“牵一发而动全身的”,因此在基础模型修复后应当及时恢复至正常运营状态。
For risk incidents that occur at the service application layer, a basic assessment should be made regarding the source of the risk. When the risk incident originates from the user side, the service provider should not only fulfill the aforementioned emergency remedial and notification obligations but also implement corresponding restrictions and punitive measures against the user. For instance, if a risk incident bubbles up from the user conducting "data poisoning" of the model, the user should be held accountable afterwards. If the risk incident does not originate from the user side, it should be traced back to higher levels to further determine whether the risk comes from the foundation model layer or the specialized model layer. This helps identify the entity responsible for post-incident response and bearing liability.
针对服务应用层发生的风险事件,应当对风险来源作出基本判断。当风险事件出自用户端,服务提供者除了履行上述应急补救义务和告知义务外,还应当对用户实施相应限制和惩罚措施。例如,因用户向模型实施“数据投毒”行为而酿成风险事件,应当事后向用户追责。当风险事件并非出自用户端,则应当向上层追溯,进一步判断风险来自基础模型层还是专业模型层,以确定事后应对义务的履行主体和责任承担主体。
The following excerpt is a translation of Linghan Zhang’s paper, titled “Legal Positioning and Tiered Governance of Generative Artificial Intelligence”(张凌寒:生成式人工智能的法律定位与分层治理).
▶ Cite Our Translation Concordia AI. “Linghan Zhang — Chinese Perspectives on AI Safety.” Chineseperspectives.ai, 29 Mar. 2024, chineseperspectives.ai/Linghan-Zhang.
▶ Cite This Work
张凌寒(2023). 生成式人工智能的法律定位与分层治理. 现代法学(04),126-141.
Translation
Generative artificial intelligence (AI) governance should adapt to the changes brought to society by technological development and reconsider how to construct a more effective governance framework after the underlying technological logic of AI governance has changed. Generative AI governance should change China’s existing regulatory system of "technology supporter—service provider—content producer" to layered regulation of "foundation model—specialized model—service application." Different levels should adapt to different regulatory approaches and tools.
原文
生成式人工智能的治理应顺应技术发展给社会生产带来的变化,重新思考人工智能治理底层技术逻辑改变后,应如何更为有效的构建治理框架。生成式人工智能的治理应改变我国原有的“技术支持者—服务提供者—内容生产者”的监管体系,实施“基础模型—专业模型—服务应用”的分层规制。不同的层次适配不同的规制思路与工具。
One reason for tiered regulation is that the distinction between “service provider” and “content producer” is meaningful only at the service application layer of generative AI. At the foundation model and specialized model layers, this distinction is not strongly related to the regulatory objective of the “content producer” concept. This is because the concept of “content producer” stems from information content security regulations, which aim to ensure compliance with bottom-line obligations for ensuring information content security for information published for the public through service providers. However, the foundation model and specialized model layers of generative AI either are trained and operated solely within companies or provide interfaces to empower vertically segmented businesses (B-side) and do not directly interact with users (C-side). To achieve the regulatory goal for "content producers," it is sufficient to impose relevant requirements at the service application layer and implement effective filtering and review. The functionalities of generative AI extend far beyond "content generation" and have become a new type of digital infrastructure. Therefore, issuing requirements for the entire industrial chain for AI as only a "content producer" based on its ability to generate text, video, and audio does not comport with its functional models.
分层规制的原因之一,在于只有在生成式人工智能的服务应用层有划分“服务提供者—内容生产者”的意义,在基础模型和专业模型层则与“内容生产者”规制目的关系不强。这是因为“内容生产者”概念来自信息内容安全监管,其制度目的在于向社会公众通过服务提供者发布信息,需遵守底线负有相关义务以保证信息内容安全。但是,生成式人工智能的基础模型层和专业模型层要么只在企业内部训练运行,要么向垂直细分领域的企业(B端)提供接口以赋能,并不直接与用户(C端)发生交互。而实现对“内容生产者”的规制目的,只需要在服务应用层提出相关要求,做好过滤审核即可。生成式人工智能的功能远不限于“内容生成”而已经成为新型数字基础设施。因此,仅仅由于其生成文本、视频、音频等一项功能就以“内容生产者”做全产业链的要求并不符合其功能业态。
A second reason for tiered regulation is to reduce the obligation to be cautious at the technical end for the foundation model and specialized model layers, in order to promote industry development. In the present governance framework, technology providers have the lowest obligations regarding information content security, while it is highest for content producers. In the early stages of internet development, service providers enjoyed years of liability exemptions, which are considered to have been crucial for the rapid development of the internet industry. Generative AI can continue to adopt the previous regulatory regime for information content security at the service application layer, achieving the governance goals for information content security. If the foundation model and specialized model layers were also subjected to the requirements for "content producers," it would become a burden on technological innovation.
分层规制的原因之二,在于减轻技术端即基础模型层和专业模型层的注意义务,促进产业发展。在现有的治理框架内,技术提供者对信息内容安全的注意义务最低,内容生产者对信息内容安全的注意义务最高。在互联网发展早期,服务提供者享受了多年的责任豁免,这也被认为是网络产业发展迅速的重要原因。生成式人工智能在服务应用层仍沿用之前信息内容安全的监管制度,即可实现信息内容安全的治理目的。如果基础模型层和专业模型层即按照“内容生产者”进行要求,则其将成为科技创新的负担。
A third reason for tiered regulation is to encourage companies to assume risk prevention obligations and legal responsibilities at different levels according to different layers of their business operations. Tiered governance also encourages companies to separately develop the foundation model layer and the service application layer, differentiating between business-to-business (B2B) and business-to-customer (B2C) models. This approach can free the foundation model layer from the responsibilities of content producers and encourage companies to separate out responsibility for the dissemination of information content into services provided for users. Even without splitting up business entities and services, companies and employees can assume corresponding responsibilities based on the level of risk occurrence.
分层规制的原因之三,在于鼓励企业根据不同的业态层次承担不同水位的风险防范义务与法律责任。分层治理也鼓励企业分别发展基础模型层和服务应用层,区分对企业和对用户模式。分层治理可以将基础模型层从内容生产者的责任中解放出来,鼓励企业将面向用户提供服务应用的传播信息内容部分切割出来单独承担责任。即使不进行主体和业务的切分,企业和工作人员也可按照风险发生等级承担相应责任。
In tiered regulation, different levels have their own governance philosophies and regulatory focuses. The foundation model layer should be development-oriented, focusing on technology ethics, training data, and model parameters. The specialized model layer should adopt a philosophy of prudent inclusion, focusing on critical domains and scenarios, sources and security of training data, and personal information protection. In this layer, a tiered and categorized system can be introduced. The service application layer should focus on information content security, orderly market competition, and protection of user rights. It should utilize existing regulatory tools while incorporating emerging tools and refining compliance and exemption systems on a timely basis, allowing room for trial and error in the development of emerging technologies. This approach would transform China's governance from more one-dimensional, scenario-based algorithm regulation, into integrated, systematic governance for generative AI that adapts to different governance objectives.
在分层规制中不同的层次有各自的治理理念与监管聚焦。基础模型层应以发展为导向,关注科技伦理、训练数据、模型参数;专业模型层应以审慎包容为理念,关注关键领域与场景,训练数据来源与安全,个人信息保护,并在此领域可引入分级分类;服务应用层则关注信息内容安全、市场竞争秩序与用户权益保护,沿用原有监管工具,并适时引入新兴监管工具、细化合规免责制度,给新兴技术发展留下试错空间。将我国从较为单一的场景的算法治理,演化为适应不同治理目标的生成型人工智能的复合型系统性治理。