Lan XUE
薛澜
About the author Dr. XUE Lan is a Cheung Kong Chair Distinguished Professor at Tsinghua University, where he serves as the Dean of the Institute for AI International Governance (I-AIIG), Dean of Schwarzman College, Director of the China Institute for Science and Technology Policy, and Co-Director of Global Institute for SDGs. His teaching and research interests include global governance, crisis management, and science, technology and innovation policy. From 2000 to 2018, he served as Associate Dean, Executive Associate Dean and Dean of the School of Public Policy and Management at Tsinghua University. Currently, he also serves as a Counsellor of the State Council, Chair of China's National Expert Committee on Next Generation AI governance, and a member of the Standing Committee of the Chinese Association of Science and Technology. Dr. Xue is a recipient of the Distinguished Young Scholar Award from National Natural Science Foundation of China, the Distinguished Contribution Award from Chinese Association for Science of Science and S&T Policy, and the Second National Award for Excellence in Innovation in China. He holds a PhD in Engineering and Public Policy from Carnegie Mellon University.
关于作者薛澜,博士生导师,清华大学文科资深教授。他担任清华大学人工智能国际治理研究院院长,清华大学苏世民书院院长,清华大学中国科技政策研究中心主任,清华大学全球可持续发展研究院联席院长等。他的研究领域包括:公共政策与公共管理,科技创新政策,危机管理及全球治理。2000-2018年,曾先后担任清华大学公共管理学院副院长,常务副院长,院长。目前,他还兼任国务院参事,国务院学位委员会公共管理学科评议组联合召集人,国家新一代人工智能治理专业委员会主任,中国科学技术协会第十届全国委员会常务委员会委员。
薛澜教授曾获国家自然科学基金委员会杰出青年基金,教育部 “长江学者” 特聘教授,中国科学学与科技政策研究会杰出贡献奖等。他于1991年获美国卡内基梅隆大学工程与公共政策博士学位。
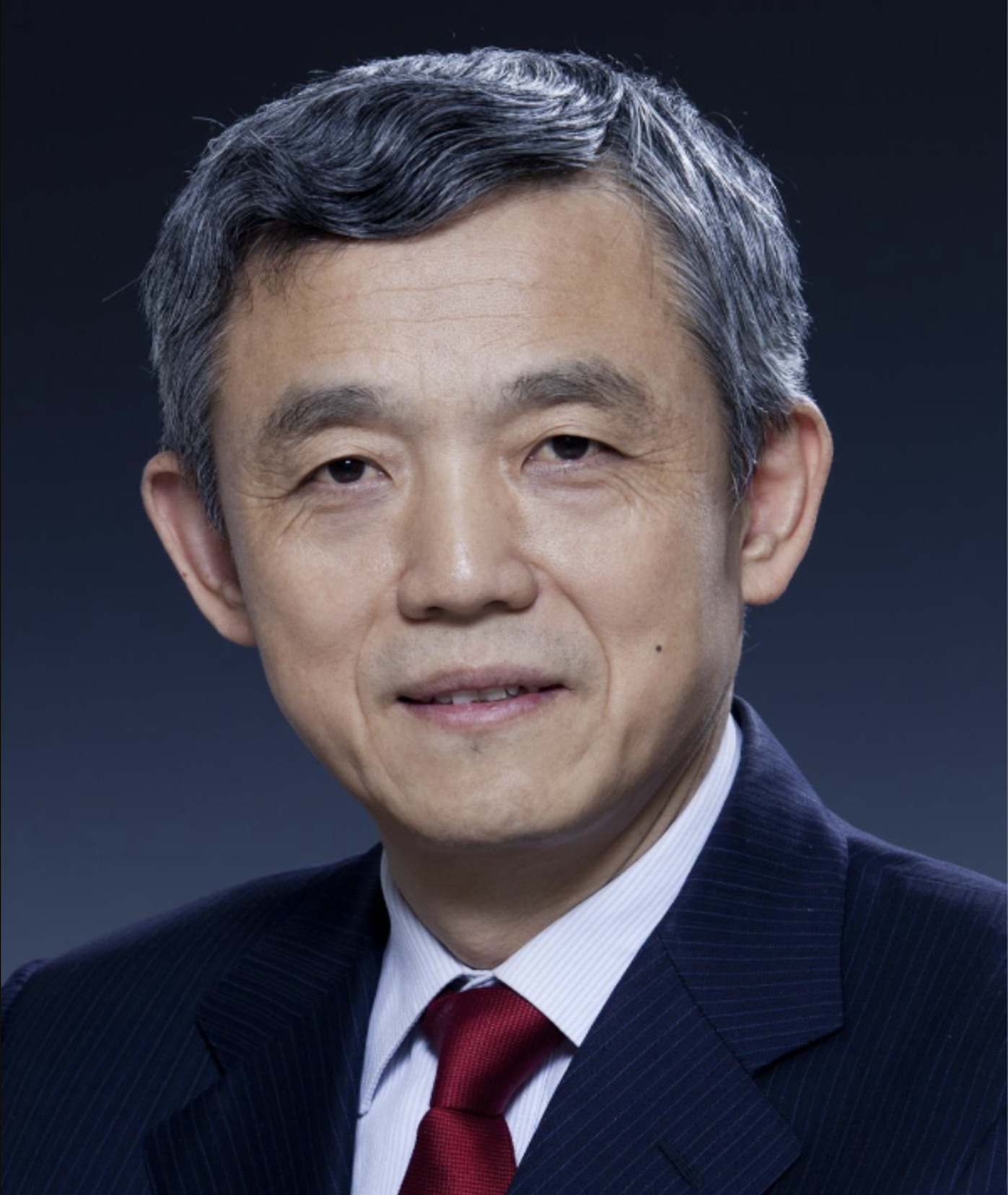
The following excerpt is a translation of Lan Xue’s interview with The Future Forum in 2021, titled “The Theory and Practice of AI for Good” (未来论坛AI伦理与治理系列01期:AI向善的理论与实践).
▶ Cite Our Translation
Concordia AI. “Lan Xue — Chinese Perspectives on AI Safety.” Chineseperspectives.ai, 29 Mar. 2024, chineseperspectives.ai/Lan-Xue.
▶ Cite This Work 薛澜(2021). “AI向善的理论与实践”. 在未来论坛AI伦理与治理系列01期的发言. https://www.163.com/dy/article/GBTR244R0512M9G9.html
Translation
With China's continued development, we have seen that in many new technological fields, China has already begun to take a leading position and started experimenting with the initial applications of those technologies. In previous Industrial Revolutions, the technologies we employed had already been used for many years in many Western countries. As a result, their various drawbacks and problems had been thoroughly researched, and they were relatively mature when applied in China. However, in the Fourth Industrial Revolution, we are also at the forefront, witnessing the emergence of many new technologies accompanied by many uncertainties. For example, technologies such as artificial intelligence (AI) and gene editing have a similar character: they have the potential to bring about substantial benefits and improve public welfare, but they could also generate potential problems and significant risks.
With China's continued development, we have seen that in many new technological fields, China has already begun to take a leading position and started experimenting with the initial applications of those technologies. In previous Industrial Revolutions, the technologies we employed had already been used for many years in many Western countries. As a result, their various drawbacks and problems had been thoroughly researched, and they were relatively mature when applied in China. However, in the Fourth Industrial Revolution, we are also at the forefront, witnessing the emergence of many new technologies accompanied by many uncertainties. For example, technologies such as artificial intelligence (AI) and gene editing have a similar character: they have the potential to bring about substantial benefits and improve public welfare, but they could also generate potential problems and significant risks.
原文
随着中国不断的发展,我们发现在很多新的技术领域,我国已经开始逐渐走在前沿,并开始尝试它的首次应用。前几次工业革命,我们用的技术都在很多西方国家已经用了很多年,所以它的各种弊病和问题也都研究得比较透彻,到中国来应用的时候应该是比较成熟的。但是到第四次工业革命,我们也已经走在前列,所以出现很多新的技术,其背后有很多不确定性。例如,人工智能技术,基因编辑等都有同样的共性:有可能给我们带来巨大的好处,改善公众的福利,也可能产生一些潜在的问题,带来巨大的风险。
随着中国不断的发展,我们发现在很多新的技术领域,我国已经开始逐渐走在前沿,并开始尝试它的首次应用。前几次工业革命,我们用的技术都在很多西方国家已经用了很多年,所以它的各种弊病和问题也都研究得比较透彻,到中国来应用的时候应该是比较成熟的。但是到第四次工业革命,我们也已经走在前列,所以出现很多新的技术,其背后有很多不确定性。例如,人工智能技术,基因编辑等都有同样的共性:有可能给我们带来巨大的好处,改善公众的福利,也可能产生一些潜在的问题,带来巨大的风险。
Therefore, seven or eight years ago, I began to pay attention to these issues. During the technological development process, we really need to focus on relevant governance issues. This way, we will avoid problems which, if neglected in the early stages, would become major issues later on. This could lead to greater resistance from the market to the technological applications, and it might even hinder technological development. Professor Guo is also an expert in this field, and we often collaborate, including the release of the Guidelines for Risk Governance of Artificial Intelligence Technology in China by the National Information Security Standardization Technical Committee (TC260) earlier this year. In June 2019, China’s AI Governance Expert Committee launched AI governance principles, aiming to provide guidance to relevant companies and researchers. Naturally, extensive discussions are still required on how to actually implement these principles or how to address these issues.
所以七、八年前我开始关注这方面的问题。我们应该在技术发展的过程中高度关注相关的治理问题,这样就能够避免这些技术发展前期不加注意,到后来真正产生大问题。因而导致市场对技术的应用产生更大的抵触,甚至可能会导致技术难以发展。郭老师也是这方面的专家,我们经常在这方面合作,包括今年年初由信安标委发布的我国人工智能技术风险治理指引。2019年6月,我国人工智能治理专家委员会推出一个人工智能治理准则,希望在这方面给相关企业以及研究者提供指导。当然,真正怎么去落实这些准则、抑或是去解决这里面的问题,还需要讨论很多。
I believe there are several aspects to consider: firstly, when technological applications bring many benefits, there may be potential negative impacts, and this requires attention and should be understood clearly. Secondly, ethical issues may arise during the application of technology. We need to carefully consider questions about whether such applications are appropriate, whether they might bring negative impacts to society or individuals, and how we can balance the pros and cons. Thirdly, technological applications may bring about many unknown risks. The other speakers are all technical experts, and we are all discussing the question of whether true artificial general intelligence (AGI), once developed, will become our master. This potential, unknown risk is fairly concerning for everyone. Finally, implementation of AI governance faces significant challenges. Governance of other technologies can be achieved through controlling specific technical indicators. For instance, the speed limit system creates limitations on the speed of cars, achieving a balance on control. However, for AI governance, we fundamentally do not know how AI makes decisions, which presents a major challenge for governance, and these issues require a high level of attention.
我认为有几个方面需要考虑:一是技术应用带来很多向善的益处时,可能存在潜在的负面影响,这一点需要关注,需要了解清楚。二是技术应用过程中有时候可能出现伦理问题,到底应用是否合适,会不会给社会或者个人带来一些负面的影响,我们怎么去权衡利弊。三是技术应用可能带来很多未知风险,其他几位都是技术专家,真正所谓通用的人工智能发展起来,会不会变成我们的主人,这也是大家都在讨论的话题。这种潜在的不知名的风险,也是大家比较担心的。最后,人工智能治理的执行也存在极大的困扰。其他技术的治理可以通过具体的技术指标调控来实现,比如汽车,可以通过限速系统(Speed limit),将开车的速度加以限制,来获得一个控制的平衡点。但是就人工智能的治理而言,我们根本不知道它怎么做决策,所以这给治理也带来很大的挑战,这些问题需要予以特别高的关注。
Moderator: AGI is a very interesting topic. I would like to take this opportunity to ask the three experts: assuming that humans achieve AGI, AI may be able to do more while becoming more capable and powerful. However, when AGI develops to a certain stage, it may pose a level of threat to humanity. In such a situation, should we really develop this technology? In most discussions, everyone tends to say that scientific and technological research should be limitless and unbounded, and we should go wherever the research leads us. But, if we anticipate certain problems to occur, should we hit the pause button? Regarding development of AGI, should there be limits, and if so, where should they be set? I would like to ask the three experts to help us answer these questions.
主持人:通用人工智能是个非常有意思的话题,我想趁这个机会问一下三位专家,假设人类实现通用人工智能以后,AI有可能做出更多更能干、更强大的事情。但是,通用人工智能发展到某个阶段,可能会对人类产生一定的威胁,在这样的情况下,我们到底应不应该开发这项技术?一般性地讨论时,大家都会说科学技术研究应该无止境、无前沿,走到哪算哪,但当我们预料到某些问题的时候是否应该按下暂停键?在通用人工智能技术开发方面,它的界限要不要设、应该设在哪儿?想请三位专家来帮我们解答一下。
Xue Lan: This question is very important. Although the United States currently views China as a major competitor in the field of AI, precisely due to the existence of such risks, the United States, China, and many other countries should closely cooperate to prevent potential attacks by malicious actors on society and the human community. This is an important foundation for our cooperation.
这个问题非常重要,虽然现在美国把中国视作人工智能领域中的主要竞争对手,但恰恰是由于这种风险的存在,美国、中国和很多其他国家都应该密切合作,以避免这些不法分子对于社会及人类共同体的潜在攻击,这一点是我们合作的重要基础。
The following excerpt is a translation of Lan Xue’s essay, titled “Promoting Agile Progress in AI Governance through Mutual Trust and Interaction“ (薛澜:推动人工智能治理在互信互动中敏捷前行)
▶ Cite Our Translation
Concordia AI. “Lan Xue — Chinese Perspectives on AI Safety.” Chineseperspectives.ai, 29 Mar. 2024, chineseperspectives.ai/Lan-Xue.
▶ Cite This Work
薛澜(2022-10-26). “推动人工智能治理在互信互动中敏捷前行”. 中国社会科学网.
Translation
Currently, an agile governance "prototype" oriented towards coordinating innovative development with governance is emerging. This involves government-led rulemaking along with the collaborative interaction of diverse entities, especially as agile thinking in the government and agile behavior in businesses are emerging. However, we must also be aware that, upon the basis of the concept and guiding principles of agile governance gaining recognition from various sectors, the specific implementation of governance mechanisms will still face multiple challenges, especially in some key dimensions guiding the formation of governance mechanisms.
原文
当前,以协调创新发展和治理为导向,由政府主导规则制定、多元主体参与协同互动的敏捷治理“雏形”正在浮现,尤其是政府敏捷思维和企业敏捷行为都在孕育中。但是我们也要意识到,在敏捷治理的概念与指导原则获得社会各界认可的基础上,治理机制的具体实现过程中仍将面临多重挑战,特别是在引导治理机制形成的一些关键维度上。
There is a gap between societal expectations and the agile actions of businesses, with a lack of agreed-upon actions in industry. Under the heavy hand of regulations, agile behavior by businesses remains stuck in technical thinking, and the nurturing of governance thinking will take time. Most companies, beneath the sword of oversight, act blindly based solely on the direction of regulators, and aim to manage risks by relying on technical solutions to reduce algorithmic and product vulnerabilities. However, the risks of AI cannot be effectively solved or anticipated if companies fail to take broader, more forward-looking actions from the perspective of multi-stakeholder governance. In the ideal agile governance model, companies should proactively engage in dialogue with regulators, contribute critical knowledge and information, engage with industry peers, and make clear that collaboration is emphasized over competition. Companies should not only achieve consensus within industry on a set of best practices among companies, using this to develop key industry standards, promoting formation of mechanisms for common learning and peer feedback; leading companies should also more actively contribute governance practices and experiences to the industry, lead the dissemination of science and technology ethics concepts, and contribute regulatory wisdom to the entire industry. Therefore, the next steps for businesses are to progress from agile norms internally within companies to connect with the industry norms system and the governmental governance system, mobilizing the entire industry for agile collaboration through practical wisdom.
企业的敏捷行动与社会期待尚有差距,缺乏来自行业的共识行动。监管重拳下,企业的敏捷行为还停留在技术思维惯性上,治理思维的培育尚需时日。多数企业在监管之剑下指哪儿打哪儿,希望以技术解决方案来实现风险管理,减少算法与产品漏洞。但倘若企业未能站在多方共治的角度上采取更广泛、前瞻的行动,人工智能的风险问题依然无法有效化解或提前研判。理想敏捷治理模式中的企业,应更为主动地与监管互动,贡献关键知识信息,与业内同行交流,明确合作大于竞争。不仅企业之间需要就一些好的做法达成行业共识,并就此推进关键行业标准的制定,促进共同学习与同行评议机制形成;而且头部企业也应更积极地向行业贡献治理实践经验,引领科技伦理理念传播,贡献全行业监管智慧。因此,从企业内部的敏捷规范,走向与行业规范体系、政府治理体系的密切衔接,依赖实践智慧动员全行业进行敏捷联动,是企业下一步的改进方向。
In negotiations around enacting oversight, the government should strike a balance between a hard and soft approach and control the rhythm. The institution and implementation of government regulatory rules are transitioning from a "one-size-fits-all" model to a "negotiation" stage. This also signifies that both sides need to rapidly interact to obtain timely feedback if they want to avoid the negotiation reducing their efficiency in innovation and development. Otherwise, the market will be afraid to innovate due to lack of guidance, and the government may revert to more simple and crude styles of regulation. Currently, mastering the pace of governance remains a challenge for regulatory agencies. The government faces the difficulty of needing to flexibly choose goals and tools to continuously tweak the direction of regulation. The first challenge is how to translate abstract governance principles into concrete laws and regulations. For example, efficiency, fairness, safety, and freedom can all be incorporated into governance goals in abstract scenarios, but the prioritization between these goals differs in different implementation scenarios. The ranking and trade-offs of diverse goals need to be based on rapid multi-party consensus. The second challenge is how to strike a balance between a heavy-handed and a light-touch approach in the selection and use of governance tools. For example, high-risk, clear violations require laws and regulations that can be clearly applied, while commercial activities that are still unclear but show signs of risk should be addressed with flexible methods. Developing government oversight that is agile and can learn requires cultivating the government's ongoing ability to understand and track industry developments, strengthening the construction of communication platforms and all sorts of research support institutions and think tanks, and emphasizing rapid follow-up in policy evaluation under an empirical paradigm.
政府的监管制定要在讨价还价中刚柔并济与把握节奏。政府监管规则的制定与实施,正在从“一刀切”的模式进入“讨价还价”阶段。这也意味着,若不想因监管双方的讨价还价而损失创新发展的效率,双方就要快速互动以获得及时反馈迭代。否则,市场将因缺乏指引不敢创新,政府也易回归到简单粗暴式规制。在当下,把握治理节奏仍然是监管部门的一个课题,政府面临必须依赖目标与工具的灵活选择以不断调整监管方向的困难。首先面对的是如何将抽象的治理原则落实为具体的法律法规。例如,效率、公平、安全、自由在抽象情景下都可被纳入治理目标,却在不同场景落实中目标优先级存异。多元目标的排序和取舍需建立在快速的多方共识上。其次,如何在治理工具的选择与使用中形成重拳与点刹的刚柔并济之道。例如,高风险、明确的违规行为需要明确适用的法律法规,而尚不清晰、存有危险迹象的商业行为,则要用柔性方法处理。培育政府持续行业理解与追踪研判能力,加强各类智库机构研究支撑与沟通平台构建,重视实证范式下政策评估工作的快速跟进,是构建政府监管敏捷学习的关键所在。
In a situation of "common ignorance," building trusting relationships is the key to agile governance. The core of creating agile governance is constructing multi-party communication and trust while facing the challenge of "common ignorance" among companies, governments, and academia due to the high level of uncertainty of technological development. In simple applications of AI technology, businesses have a clearer understanding of the risk boundaries of technology and can continuously provide rules for business models within the regulatory framework. However, with the rapid updating and iteration of data and algorithms, there will be a state of "common ignorance" involving both businesses and regulators in a certain sense. Given the enormous uncertainties of innovation and risk, building trusted channels among multiple parties and maintaining smooth communication mechanisms are crucial. At the same time, actual implementation of a diversified, common governance system cannot ignore participation and guidance from the societal dimension. For example, balancing the relationship between societal opinion and technological development, preparing social risk contingency plans, and fostering emergency management thinking are critical. In addition, in discussions on AI ethics and governance, it is essential to both realize the critical role of scientific research institutions as trusted intermediaries and while also being aware that relevant discussions are not just the "privilege" of experts and scholars; all of society may have different views on AI governance issues and advocate for diverse governance ideas–and indeed they have this responsibility.
在“共同无知”下,信任关系构建是敏捷治理的关键。面对技术发展高度不确定性导致企业、政府、学界的“共同无知”难题,构建多方的交流与信任是敏捷治理形成的核心。人工智能技术简单应用条件下,企业较为清楚技术的风险边界,并可以在监管框架下不断规范商业模式。但随着数据与算法的快速更新迭代,在某种意义上会出现企业方与监管方的“共同无知”情形。创新与风险的巨大不确定性使得搭建多方信任桥梁,畅通交流机制异常重要。同时,多元共治体系的真正落实还不能忽略社会维度的参与和引导。例如,平衡社会舆情与技术发展之间的关系,着手社会风险预案准备与应急管理思维培育等,都是个中关键。此外,在人工智能伦理与治理的讨论中,既要意识到科研机构作为信任中间人的关键角色,也要意识到相关讨论不只是专家学者的“特权”,全社会都有可能、也有责任对人工智能的治理问题有不同看法,提供多元治理思想的倡导。
In the field of AI, we really hope to achieve an agile governance model oriented towards multi-stakeholder collaboration. Through industry coordination, government learning, and trust-building efforts, we can form a robust, trusted governance relationship, shifting from "common ignorance" to jointly addressing complex governance demands, from "negotiation" to advancing hand-in-hand with mutual trust and interaction.
在人工智能领域,我们特别希望实现迈向多主体合作的敏捷治理模式,通过行业联动、政府学习、信任构建的努力,形成坚固的信任治理关系,从“共同无知”到联手应对复杂的治理需求,从“讨价还价”变为互信互动中携手前行。
Translator’s notes
1. Professor GUO Rui (郭锐) at Renmin University of China was another guest at this event. Note that the name of the TC260 document referenced by Professor Xue appears to be AI Ethics and Safety Risks Prevention Guide (人工智能伦理安全风险防范指引).